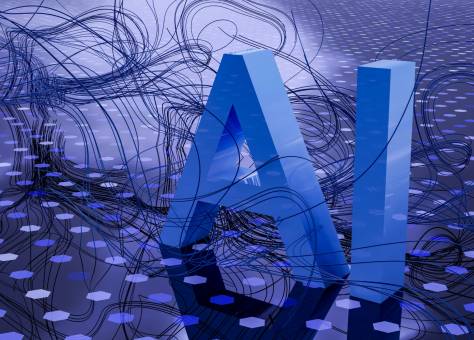
AI power: Expanding data center capacity to meet growing demand
McKinsey & Company recently wrote about the soaring demand for AI-ready data centers and the opportunity this has for companies and investors across the value chain. McKinsey suggests that how quickly they grasp them could determine the pace at which AI is deployed.
McKinsey wrote: The race is on to build sufficient data center capacity to support a massive acceleration in the use of AI. Data center demand has already soared in response to the role data plays in modern lives. But with the emergence of generative AI (gen AI), demand is set to rise even higher. And that is likely to presage a supply deficit.
As challenging as this could be, companies and investors along the entire data center value chain have an opportunity to help address the looming capacity crunch—if they understand the requirements of data centers designed for the AI age. A big chunk of growing demand—about 70 percent at the midpoint of McKinsey’s range of possible scenarios—is for data centers equipped to host advanced-AI workloads. And the nature of those workloads is rapidly transforming where and how data centers are being designed and operated.
Exploding demand and lagging supply
Future demand for data center capacity will depend on factors that are still hard to accurately determine. The pace of adoption of advanced-AI use cases will certainly count, but so too will the mix of different types of chips deployed and their associated power consumption, as well as the balance between cloud and edge computing for AI workloads and the typical compute, storage, and network needs of AI workloads. This explains McKinsey’s range of estimates. Our analysis of current trends suggests that global demand for data center capacity could rise at an annual rate of between 19 and 22 percent from 2023 to 2030 to reach an annual demand of 171 to 219 gigawatts (GW). A less likely yet still possible scenario sees demand rising by 27 percent to reach 298 GW. This contrasts with the current demand of 60 GW, raising the potential for a significant supply deficit. To avoid a deficit, at least twice the data center capacity built since 2000 would have to be built in less than a quarter of the time.
However, estimating the precise size of that deficit is hard because of uncertainties surrounding the pace of rising demand, the extent to which innovations might improve power efficiency, and limited knowledge concerning the longer-term expansion plans of data center owners and operators. But even if all currently known plans are delivered on time, there could still be a data center supply deficit of more than 15 GW in the United States alone by 2030.
Demand for AI-ready capacity is the main driver of this potential deficit—as it must provide the high computational power and power density required by AI workloads. Our analysis suggests that demand for AI-ready data center capacity will rise at an average rate of 33 percent a year between 2023 and 2030 in a midrange scenario. This means that around 70 percent of total demand for data center capacity will be for data centers equipped to host advanced-AI workloads by 2030. Gen AI, currently the fastest-growing advanced-AI use case, will account for around 40 percent of the total.
New location, design, and operational requirements
Data centers have seen steady changes and improvements over the past decade, gradually getting bigger, housing more power-hungry, high-density servers, and operating more efficiently and sustainably.
AI has forced the pace of progress, however. Most notably, data centers have exploded in size in terms of power consumption. Ten years ago, a 30-megawatt (MW) center was considered large. Today, a 200-MW facility is considered normal. The driving force for this is the computing power required for AI workloads, which, in turn, bumps up energy consumption.
Data center location and power infrastructure
As more data centers are built and the amount of power they require grows, power supply is becoming an issue in markets that have traditionally attracted clusters of data centers, such as Northern Virginia and Santa Clara in the United States. Many utilities find they haven’t been able to build out transmission infrastructure quickly enough, and there is concern that at some stage they may be unable to generate sufficient power.
This can slow data center expansion. For example, some utilities initially offer only relatively small chunks of power to data centers, which they then increase as they build out the power infrastructure—perhaps in 15- to 25-MW tranches for a 100-MW new data center campus. Additionally, in some countries, concern about the pressure data centers exert on electricity grids as well as the impact on national climate targets have brought a complete halt to the building of new ones. Ireland, for instance, has stopped issuing new grid connections to data centers in the Dublin area until 2028. Ireland’s transmission system operator estimates that data centers will account for 28 percent of the country’s power use by 2031.
The fact that not all AI workloads are equal has partly alleviated the power problem. Historically low latency has been one of several critical factors in determining data center location, often leading colocation providers to establish facilities near population centers. When AI models are being trained, typical performance factors such as low latency and network redundancy are less important. It is only when the model is put into operation—during the inferencing workload—that these factors become crucial for optimal performance. Hence, data centers dedicated to training AI models are being built in more remote locations in the United States, such as Indiana, Iowa, and Wyoming, where power is still abundant and grids are less strained (Exhibit 4). But given the lack of adequate power transmission infrastructure in these locations, power supply may still become an issue as demand grows.
Demand for AI-ready data centers is surging, and with it the potential for a serious supply deficit. The extent to which companies and investors in the value chain are able to speed the build-out of those centers could determine their fortunes. And ultimately, it could determine the pace at which AI is deployed in all our lives.
Read the full article here: https://www.mckinsey.com/industries/technology-media-and-telecommunications/our-insights/ai-power-expanding-data-center-capacity-to-meet-growing-demand